What opportunities and risks does AI present for climate action?
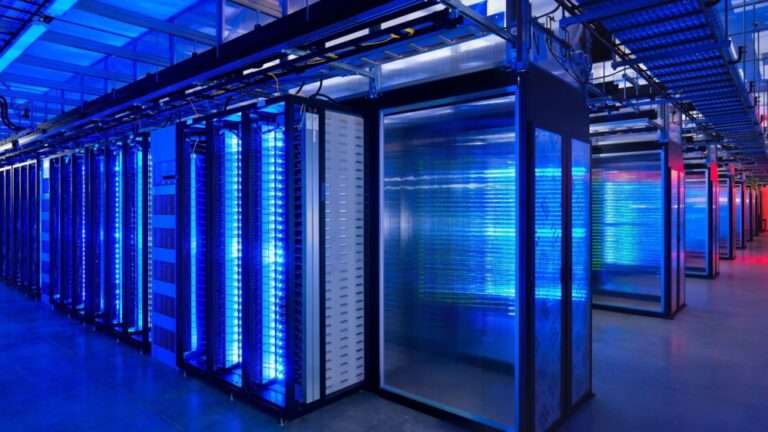
The net zero transition and the digital transformation are the two most powerful forces of the 21st century. There are promising signs that artificial intelligence (AI) can support climate action, but at the same time, it carries risks: AI is energy-intensive to run and the ways in which it is applied could exacerbate social and economic inequalities. These economic and technological transformations need to be harnessed in a way that supports just, fair and inclusive economic development.
What promise does AI hold for combatting climate change?
One of the frequently cited barriers to accelerating action on climate change is the size and complexity of the datasets, variables, models and scenarios involved. AI and its close cousin machine learning (ML) – a subfield of AI that identifies patterns to support the completion of specific tasks – are revolutionising our capacity to gather, complete and interpret large, complex and disparate datasets, making it possible to enhance data-driven decision-making for climate action. AI can be used in climate research and modelling; in financial markets (e.g. to forecast carbon prices); and in education and behavioural change, (e.g. using data on behavioural patterns to ‘nudge’ people to choose a more sustainable option, or to calculate the carbon footprint of products to help individuals make informed decisions).
Organisations are increasingly focusing on the intersections of climate and data and are exploring the potential applications of AI and ML. For example, in 2020 Refinitiv and the World Economic Forum launched the Future of Sustainable Data Alliance at Davos, and the Network of Central Banks and Supervisors for Greening the Financial System (NGFS) launched a workstream on bridging data gaps a few months later.
AI can be used to accelerate progress towards a low-carbon economy by supporting efforts to mitigate carbon emissions across sectors including energy and transport, and to develop carbon removal systems. Its main application is in gathering and completing complex datasets on emissions and their climate effects, thereby helping to model policy and climate-risk analysis and optimise processes and supply chains.
In energy, AI can help to manage predictability in the grid and thereby minimise emissions from standby generators that are fired up when energy demand outstrips supply. The intermittent nature of renewables, combined with the difficulty of forecasting supply and demand, is one major hurdle to overcome in the clean energy transition. ML can help create electricity markets with advanced forecasting, scheduling and control capabilities that can manage both variable electricity production and flexible demand through more efficient, real-time balancing in the grid. DeepMind has demonstrated that such applications can help increase the economic value of wind energy by 20% by reducing reliance on battery storage and other sources of power on standby. Similar applications are being developed for solar. ML can also be applied to reduce personal energy consumption and to quickly assess the energy consumption of buildings – and even entire cities – in real time.
In transport, ML is being applied to optimise route planning, which in turn helps to cut the number of miles travelled by vehicles, reducing emissions. AI can be applied to help build intelligent transport systems through better modelling of demand and by planning new infrastructure in ways that discourage urban sprawl, limiting the length of journeys that people need to take. It is also being used to better monitor traffic in existing systems through automated computer vision. On a smaller scale, ML is contributing to the development of autonomous vehicles and is playing an integral part in the development of shared mobility services, again with the effect of reducing emissions from car journeys.
Additionally, AI can support climate change mitigation efforts through carbon removal, both technological (e.g. assessing carbon capture and storage sites) and environmental methods (i.e. carbon sinks such as forests). In the case of forest carbon sinks, the Multi-Mission Algorithm and Analysis Platform being developed by NASA and the European Space Agency uses AI in the analysis of varied datasets such as satellite instruments, the International Space Station, and airborne and ground sources to help monitor forest biomass and trends in the absorption and release of carbon.
How can AI support adaptation, resilience and biodiversity conservation?
One major application of AI for climate adaptation and resilience is in hazard forecasting and improving climate disaster alert systems. Deep-learning algorithms can improve the sensitivity and specificity of early warning systems about climate tipping points. This can be used to project long-term trends in a particular region (e.g. modelling sea-level rise) to help people prepare for major climate change-induced changes. Researchers at the British Antarctic Survey and the Alan Turing Institute have developed IceNet, a predictive tool using AI that forecasts changes in sea ice levels using data from satellite sensors in climate modelling.
AI-powered early warning systems can also help humans better respond to extreme weather events such as cyclones and determine with greater accuracy the location and extent of near-term predicted storms, wildfires and floods. For example, AI models based on satellite imagery can help show the real-time location of wildlife boundaries on Google Maps and assist firefighting operations in the US, Canada, Mexico and Australia. The Google Flood Hub uses machine learning models to issue detailed alerts and in 2021 sent 115 million flood alert notifications to 23 million people across the world.
Also using satellite technology, AI can help to track biodiversity loss after forest fires and estimate the water content in the tree canopy in combination with drought forecasting to help predict which regions are most at risk. Following a climate-related disaster, ML can also be used to analyse satellite imagery to identify which areas need help. More broadly, ML can support biodiversity conservation by increasing the efficiency with which we monitor ecosystems, replacing traditional, manual ‘on-the-ground’ observation methods. Aerial imagery can help automatically track tree cover, while remote sensing data and image-based sensors can help infer biodiversity counts. Camera traps can take photos automatically whenever a motion sensor is activated, and computer vision can be used to classify the species that pass by, supporting a real-time and less labour-intensive species count. In monitoring ocean life, marine robots can survey large areas underwater while ML can be used to identify plankton automatically from underwater cameras and estimate fish populations from the structure of coral reefs. Beyond data collection, AI-powered analysis of large-scale simulations can facilitate tracking of how ecosystems will evolve across potential future climate scenarios.
Agriculture is another major area in which AI can support adaptation and resilience. Applications include software and hardware tools for precision agriculture and intelligent irrigation AI tools that enable farmers to grow crops with significantly less water. X’s Project Mineral has developed a low-emission electric power rover fitted with solar panels that uses GPS software to identify the location of plants in the field and then applies machine perception tools to analyse the data and identify the resilience and productivity of crops in different environments, with climate change taken into account.
What are the possible drawbacks of AI for climate change?
AI has significant potential to enhance our abilities to address climate change, but it can come at a cost. The risks of AI for climate action can be grouped into three broad themes: the carbon footprint of AI itself; the environmental impact of the materials required to manufacture the technologies; and the unequal distribution of its benefits, which could further exacerbate inequalities.
AI relies on energy-intensive computers. Although each new generation of processors can usually carry out more computations with less power, and investment in the sector is driving further improvements in power and efficiency, the constant upgrading of models and retiring of old ones means the sector has a large overall life-cycle impact on climate and the environment. Many AI technologies also require rare critical minerals and resources such as cobalt, lithium and tantalum for their manufacture, whose extraction comes at a cost to the environment and local communities.
The unequal application of AI has the potential to further exacerbate inequalities between richer and poor countries globally. AI research today is predominantly led by research institutions and companies based in developed countries. This could lead to blind spots and neglect the needs of developing countries – where applications of AI for climate solutions are most urgently needed. To address this, AI solutions need to receive much more support than they currently do to ensure that the needs of developing countries are adequately addressed in terms of capital investment and capacity-building for policymakers, regulators and practitioners.
This Explainer was written by Danae Kyriakopoulou, with review by Roberta Pierfederici.