What do economic models tell us?
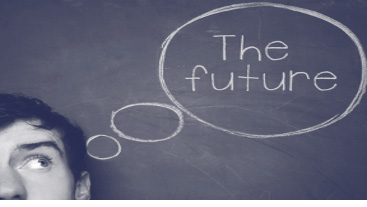
“It is difficult to make predictions, especially about the future” – so goes the saying. The same applies to economic modelling. This is not just the trite point that it is hard to make predictions about things that are uncertain, though you’d be surprised how many economic models are expressed as deterministic, that is, without probability bounds. Pindyck makes the valid point that key inputs are often chosen arbitrarily; even the best model spits rubbish out if you pump rubbish in . But I want to focus on the slightly more subtle point that the very things that are most interesting when it comes to making predictions decades ahead are those which are hardest to model. The result is that more often than not, they are simply not modelled and consequently the models tell us little about how the future will evolve and still less about the true costs and benefits of long run policies such as those to promote renewable technologies and resource efficiency.
An economic model is essentially a simplified framework for describing the workings of the economy. It exerts the discipline of forcing the modeller to formally articulate assumptions and tease out relationships behind those assumptions. Models are used for two main purposes: simulating (e.g. how would the world change relative to some counterfactual if we assume a change in this or that variable) and forecasting (e.g. what the world might look like in 2030). Economic models are great tools for simulations – given what we know about the behavioural workings of the economy, and taking these mostly as given, how might the economy respond to, say, an energy price spike? But models are much less effective at providing forecasts precisely not least because when making forecasts, very little can be taken as given. The further out the forecast, the larger the structural uncertainties making model projections at best illustrative, especially when trying to forecast the impact of non-marginal impulses such as climate change impacts or the transformation of the global energy system.
Models used by finance ministries, banks and central banks take the underlying structure of the economy as given and analyse perturbations on the margins through estimated behavioural equations. Both estimated ‘new Keynesian’ and computable general equilibrium models rely on assumptions about pre-determined long term trends or ‘convexity’ associated with diminishing marginal returns and diminishing marginal products, in order to converge on a steady state. Because they rarely look forward beyond a four year horizon, such simplifying assumptions make for good approximations of reality. In fact at the Treasury, we fixed the main forecast variables (GDP growth, unemployment, trade balances, inflation, etc…) first and then ran the model . This is actually the norm for macroeconomic forecasting – the model is essentially used as a consistency check and not a source of projections. Provided the forecast residuals are in line with past patterns, then fixing the forecast path beforehand is validated because the projections are compatible with past estimated behaviour. But looking further out, the uncertainties grow and so do the chances that structural breaks push the economy onto new paths driven by new technologies, institutions and behaviours. Characterising key variables, like output, as reverting to a deterministic mean is convenient but unrealistic the further out you look.
This causes problems for economic forecasts tasked with examining the impact of large transformative change such as transitioning to a resource efficient global economy over longer periods. The requirement that a model tends towards a steady-state equilibrium means many key dynamics are modelled as tendencies towards that equilibrium, rather than determinants of it. ‘Change’ and heterogeneity are modelled as transient states. Yet the real world is what economists call endogenous – that is, subject to systemic changes that originate within the system. Heterogeneous processes breed feedback loops which become permanent features of the system, requiring a theory of the long run characterised by such processes. Economic factors that are subject to economies of scale, capital and institutional lock in, irreversibilities, new networks and path-dependencies are hard to estimate empirically (in some cases they have never happened, yet) and even harder to model because of the non-linear dynamics. Shocks will have persistent effects (think 9/11), policy choices will have large and amplified implications (think defence spending and the internet) making prediction increasingly difficult. Different meteorological models and forecast runs make consistent and accurate global forecasts over a two week period, but then start to diverge because of the infamous ‘butterfly wing’ effect. Beyond a month or so, such forecasts diverge wildly and are considered next to useless. The same is true with economic models over long periods.
Take some real world examples. Investing in renewable energy technologies pushes their price down as a result of experimentation and learning from mistakes; so-called “learning-by-doing”. These price falls then make the investment increasingly attractive relative to conventional technologies where the gains from additional learning or scaling are smaller. As costs come down, investment increases and engineers learn how to cheaply install, connect and repair the technology (one reason why solar PV is considerably cheaper in Germany than in the US), planning institutions are updated and new networks are built or transfigured. Consumers change behaviour and demand efficiency, recycling and pedestrianisation.
Very quickly, a region can switch from one technology network to another as learning and experience makes it more attractive than the incumbent. But such inherently bi-polar path-dependent dynamics are hard—if not impossible—to model. Cities planned on a model of dense development with integrated public transport become many orders of magnitude less resource intensive than cities based on a sprawling car based model, despite having the same levels of income. Once built, they are hard to change retrospectively as behaviours and infrastructures become locked-in. Constituencies lobby for lower petrol prices and more highway lanes in the sprawling city and cycling lanes, public transport and congestion charging in the dense efficient one. The decisions made by planners in China, India and elsewhere will go a long way to determining the efficiency and resource-security of their economies as a whole. They also create sizable new markets which stimulate innovators and investors across the world.
But none of this is incorporated into standard models because the full interaction of an endogenous system is fiendishly complex to replicate and any error spreads through the model like a malignant disease. Modelling therefore requires abstraction. Not all variables can be included and not all causal processes simulated. But abstracting is fine until you abstract from the key properties of the system and then purport to forecast that system as whole. In most models, innovation which drives long term economic prospects is assumed to just happen and key features of capitalism such as the tendency towards oligopoly are assumed away in favour of the more tractable assumption of competition.
Malthus’s mistake was famously to take the structure of the global economy as given. His model assumed that technologies and processes would remain unchanged, such that the world would run low on resources in the face of growing population and demand. In fact, every extra human mouth came with a human brain. And it was human innovation that allowed agricultural yields to rocket and industrialisation to provide an unprecedented array of consumer possibilities. Modelling innovation requires understanding the unintended consequences which result from knowledge spills-overs from one sector to another. Mariana Mazzucatto tells a compelling story of how almost all the radical technologies behind the iPhone were funded by government, mostly through defence research funds: this includes the internet, GPS, touchscreen display, and even the new voice-activated Siri personal assistant . These underlying dynamics are known and predictable as processes, but not in terms of specific outcomes. Consequently, almost all models abstract these key relationships away.
There are also a number of behavioural dynamics to account for. For example, global collective action is subject to gaming where the pay-offs to individual agent’s action (countries, regions, businesses) depends on how others act. If enough players act, a critical mass or ‘tipping set’ is reached where it pays to act also. For example it may pay for a firm or economy to delay the cost of resource efficient investment, but as others make the investment, it may increasingly struggle to sell into new markets where efficiency standards improve (as was the case with US car manufacturers). Such dynamics can be modelled, but are hard to incorporate into existing integrated models. Who could have deterministically modelled the opening up of world trade in the second half of the last century? Yet these underlying dynamics are known and predictable and ultimately it is these that will shape the world.
The potential benefits from policy which directs structural change will ultimately dwarf the first round distortionary costs associated with a carbon tax here, or a new standard for efficiency there. As with defence spending, a concerted effort to push green R&D is likely to have multiplied impacts on innovation across the economy. This means that for policymakers, planning a competitive and resource-efficient economy fit for a challenged twenty-first century might make a more important priority than figuring out the short run cost of a set renewables, relevant though that is. Yet models focus almost exclusively on narrow questions of the latter type, just because they can, generating a set of costs for each action, with little understanding of the full potential benefits. The resulting predisposition towards postponing action short-circuits the more pertinent questions of how structural change can be brought about in a transparent, market-friendly manner; one which promotes competition and growth and limits the scope for rent-seeking by vested interests.
Does this mean we should jettison economic models? Absolutely not. We should still try to estimate and model known complex processes. Models are essential tools in helping us formulate, examine and understand interactive relationships. Yet while integrated economic models applied to the long term have produced valuable insights, they were never designed to serve as estimates of the total impacts of things like policies to reduce emissions and improve resource efficiency. Fully integrated endogenous systems make modelling long periods very hard because even small errors persist and explode and alter the outputs of the model like a malignant disease. Models attempt to get around this problem by assuming that key parts of that story are predetermined, yet this makes them no more realistic. What is required is a coherent theory behind long run processes of systemic change and these are best modelled separately, and not as part of a fully integrated model which imparts false notions of determinism and precision. Models are not the whole story; they are merely supporting sentences to the story and must be understood and treated as such. In a sufficiently complex system it may actually be easier to drive change than to predict it.
Dimitri Zenghelis is Co-head Policy at the Grantham Research Institute at the LSE and was previously Head of Economic Forecasting at HM Treasury. This article was originally published in Business Green on the 18 September 2013.