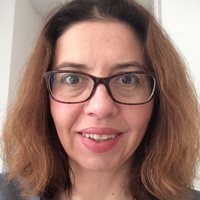
My teaching philosophy is sharing
I come from a family of teachers and from a young age I aspired to follow my father’s steps, who would bring purpose, moral, and a touch of magic in every topic he was teaching. My path brought me again to LSE, that has a magic of its own, where I can share with my students, my experience, knowledge and indeed love for statistics, and by doing so, help them achieve their dreams and aspirations. The idea of sharing was also the drive to introducing the GTA mentoring scheme, where teaching staff can share experiences, tips and assist one another in improving and developing their skills.
Statistics and mathematics are not just numbers but logic
The most common advice I give to my students is this. Break the problem into small components, visualise how the different parts are connected and follow logical steps to navigate your way towards the final answer. If you can’t solve a difficult problem, solve a simpler one. Ideally, solve many simpler problems. Practice, practice and more practice. You will soon decipher the logic behind the formulae and before long you will have surpassed your target.
As a teacher, witnessing my students’ progress from novices to masters is my best reward.
Statistics is not only relevant but essential in life
During my first class, I challenge my students to find a topic where statistics is not used, or to think how many hours ago they came across a statistical result (inadvertently or not). Throughout my teaching, I try to show the connection between the syllabus material and real life statistical applications. The deeper one understands the former, the broader the scope of the latter. In a rapidly changing work space, statistics has a prominent place. Teaching is not simply showing students the solution to existing problems but mainly inspiring them to invent new problems and quest for innovative solutions.
LSE, is an exciting pool of diverse talents, backgrounds and dreams, and teachers and students together have the opportunity to take part in shaping a bright future.
Teaching is itself a learning experience
Every class teacher goes through a similar process, that includes preparing and presenting a topic in front of an audience. It is natural to feel nervous at first, but with every class the confidence increases. Below are a few tips to make this process easier and more efficient.
1. Go through the same thought process as the students, by solving the problem independently and using first principles.
2. Do a rehearsal of the key points and have a plan, it provides reassurance.
3. Spend a few minutes in the beginning summarising the relevant theory. This makes the connection between lecture and class and helps establish continuity.
4. Give references to real life applications of the topic covered.
5. Do not feel embarrassed when you make a mistake, everyone does, it’s part of the learning process, just thank the student who spotted it.
6. Explain the logic of a formula or a proof, and feel free to skip some of the detailed calculations. Break the question into a small number of logical steps and emphasise the logic behind the solution.
7. Alternate the questions you discuss between homework and unseen. This increases the student involvement and participation.
8. Finish the class by emphasising the importance of the material covered during the class.
9. You are strongly encouraged to attend a class of a more experienced class teacher or lecturer.
Teaching improves important interpersonal skills, like presentation, assertiveness, communication and listening that are extremely useful both inside and outside the classroom.