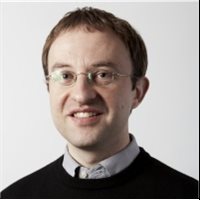
Biography - Piotr Fryzlewicz is a professor of statistics in the Department of Statistics at the London School of Economics. He has previously worked at Winton Capital Management, the University of Bristol and Imperial College London. His research interests are in multiscale modelling and estimation, time series, change-point detection, high-dimensional statistical inference and statistical learning. He is a former Joint Editor of the Journal of the Royal Statistical Society Series B.
Title - Multiscale thinking in data analysis, recursive algorithms, and data-adaptive change-point detection.
Abstract - The talk starts on a general note: we first attempt to define a "multiscale" method / algorithm as a recursive program acting on a dataset in a suitable way. Wavelet transformations, unbalanced wavelet transformations and binary segmentation are all examples of multiscale methods in this sense. Using the example of binary segmentation, we illustrate the benefits of the recursive formulation of multiscale algorithms from the software implementation and theoretical tractability viewpoints.
We then turn more specific and study the canonical problem of a-posteriori detection of multiple change-points in the mean of a piecewise-constant signal observed with noise. Even in this simple set-up, many publicly available state-of-the-art methods struggle for certain classes of signals. In particular, this misperformance is observed in methods that work by minimising a "fit to the data plus a penalty" criterion, the reason being that it is challenging to think of a penalty that works well over a wide range of signal classes. To overcome this issue, we propose a new approach whereby methods "learn" from the data as they proceed, and, as a result, operate differently for different signal classes. As an example of this approach, we revisit our earlier change-point detection algorithm, Wild Binary Segmentation, and make it data-adaptive by equipping it with a recursive mechanism for deciding "on the fly" how many sub-samples of the input data to draw, and where to draw them. This is in contrast to the original Wild Binary Segmentation, which is not recursive. We show that this significantly improves the algorithm particularly for signals with frequent change-points.