The LSE Data Science Institute (DSI) is proud to highlight the work of LSE students such as Adriana Svitkova and Idil Balci, who have published this blogpost that considers machine learning, artificial intelligence and artificial neural networks
Share your research with the DSI. LSE students can get in touch here.
Deep neural networks and the rise of the ‘AI Gaydar’
Adriana Svitkova and Idil Balci
Introduction
Physiognomy is the practice of using facial features to determine one’s character, including gender, sexual orientation, political affiliation, or criminal status (Jenkinson, 1997). For example, a strong jawline is sometimes linked to being dominant or assertive. You can imagine how dangerous this affiliation on the basis of visual cues may be — despite being around for centuries, physiognomy is now widely rejected as “a mix of superstition and racism concealed as science” (Jenkinson, 1997, p.2).
In 2017, Wang & Kosinski from Stanford University published a highly controversial paper which proposed that machine learning tools can predict sexual orientation from facial characteristics, using deep neural networks. They argued that their findings supported the prenatal hormone theory, which suggests our sexuality is determined by hormone exposure in the womb (Resnick, 2018). The paper is considered highly controversial and remains debated outside academic circles, with many critics claiming that it draws “populism” into science (Mather, 2017, cited in Miller, 2018).
Study Purpose
What is machine learning?
Artificial intelligence (AI) is the use of machines for tasks that generally require human intelligence. Machine learning (ML) is a subset of the field, training machines to automatically produce outputs on unseen data (Warden, 2011). Whilst statistics are generally used to identify underlying processes, ML is used “to predict or classify with the most accuracy” (Schutt & O’Neil, 2013).
What are artificial neural networks?
Artificial neural networks are algorithms inspired by the human brain (Marr, 2018). There will be different nodes (similar to neurons in our brain) that are stimulated by information.
What is deep learning?
“Deep” learning refers to the depth of layers in a neural network, with more than three layers typically considered a deep learning algorithm (Kavlakoglu, 2020). Deep neural networks (DNN) have more ‘hidden’ layers with their own activation function, allowing them to process and structure large amounts of data (LeCun, Bengio & Hinton, 2015). Deep learning differs from conventional ML as it requires less human intervention and is capable of ingesting unstructured data (Kavlakoglu, 2020). They are increasingly outperforming humans in tasks such as image classification, diagnosing cancer and facial recognition (Esteva et al, 2017).
Hard to follow? Imagine the order as Russian dolls. Essentially, each subfield is formed in relation to the other.
AI vs Machine Learning: What’s the Difference? (Kavlakoglu, 2020)
Data and Findings
Since most ML algorithms cannot process image data directly, they must first be converted to numbers (features). In this study, the authors use a pre-trained VGG-face classifier to convert their images into numeric data (the independent variables) and the sample’s self-reported sexual orientation divided into two — gay/heterosexual as their dependent variable (Wang & Kosinski, 2017). Their experiment is a case of supervised learning, as a predetermined set of dependent variables are used to train the algorithm to predict Y given X (Schutt & O’Neil, 2013). Due to the computational cost of training large models, data scientists commonly use pre-trained models to save time and money (Marcelino, 2018).
The authors initially gathered 130,741 images from a U.S dating website. They used the face-detection software, Face++, to locate different areas of the face (see Figure 1) and used individual profile descriptions stating one’s sexuality as the dependent variables. The dependent variables (one's sexuality) was extracted from individuals’ profile descriptions on an online dating website, where they stated who they were interested in. As you can imagine, this might not always be an apt description of one’s sexuality. The authors stated that in order to prevent bias, the features extracted were the location of the face in the image, outlines of its elements, and the head’s orientation (Wang and Kosinski, 2018; Scherfner et al., 2022).
Graphical illustration of the illustration produced by Face ++
The deep-learning algorithm used in the Wang and Kosinski (2018) research — Face++ — runs on a graph detection system and fuzzy image search technology (RecFaces, 2021). Face ++ can detect up to 106 different data points located on an individual’s face (Ibid). It operates on a network of big data — MegEngine — owned by a Chinese technology company Megvii (RecFaces, 2021; Scherfner et al., 2022). The values rendered by the Face++ algorithm were entered into a binary cross-validated logistic regression feature-based model (homosexual-heterosexual).
The authors claim that their algorithm was able to identify a person's sexuality 81% of the time. Thus, they concluded that the algorithm outperformed human judgement; there must be facial variations that reveal sexual orientation undetectable by the human eye (Sandelson, 2018). Moreover, the authors argued that the results “provide strong support” for the prenatal hormone theory, which states that the level of exposure to prenatal androgens determine our sexual orientation (Venton, 2018). They found, based on their algorithm, that gay men tend to have more feminine facial structures compared to heterosexual men, with lesbian women having more masculine features (see Figure 4).
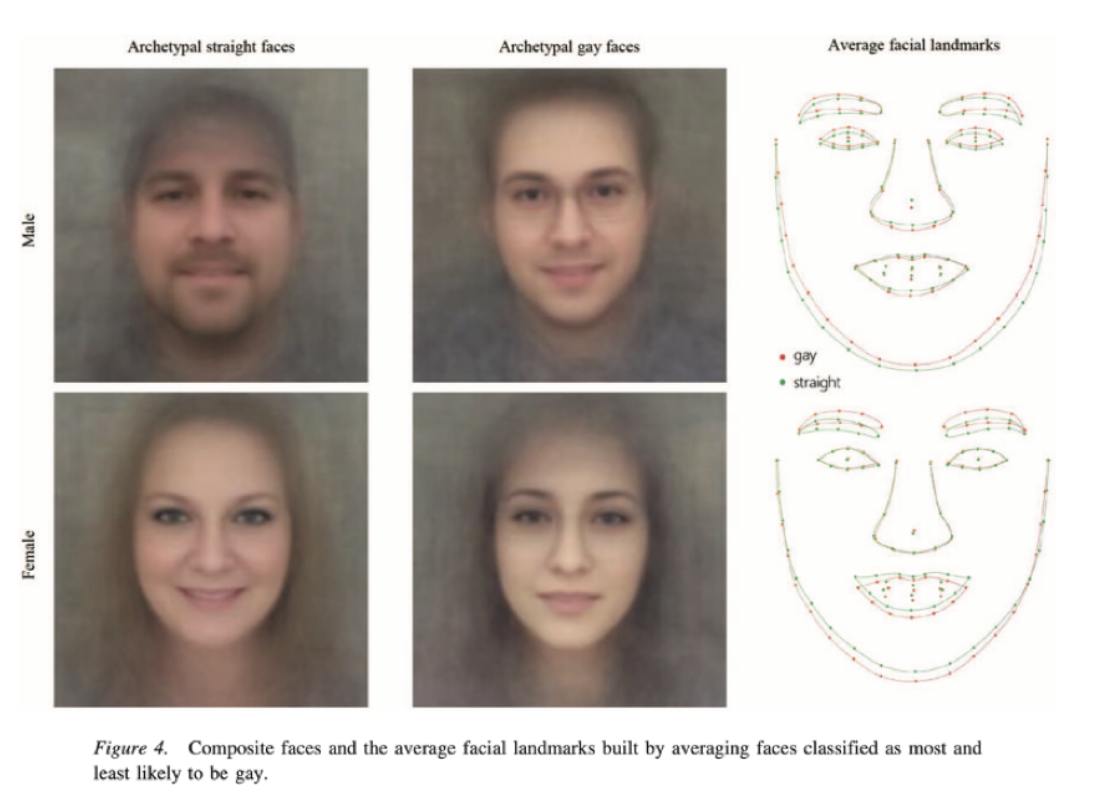
Wang and Kosinski (2018) used a database which identified faces as either homosexual or heterosexual. A similar study conducted by Wu et al. (2020) included a larger spectrum of the LGBTQ+ population and extended the bi-variate classifier, which resulted in almost half the accuracy of the classification algorithm. The major problem in the Wang and Kosinski (2018) paper is the lack of diversity in their input data; not only did they use a simple binary classifier (homosexual-heterosexual), but they also focused only on white, Caucasian individuals. This excludes trasngender, non-binary and people of colour entirely from their sample. Moreover, the human judges who were employed by the survey to represent human judgement were AMT workers. However, AMT workers tend to be male (70%), and thus, even the human judgement sample used was not fully representative of society (Martin et al., 2017).
Implications
Since its publication, the prospects of an ‘AI gaydar’ has gained much criticism. Despite being dismissed as ‘fake science’, the 2017 study draws our attention to the growing use of facial recognition software (Lior, 2018). Sometimes, this may have a positive impact. Consider Apple’s face ID for passwords. Could we imagine a world today without such convenience or efficiency? From a business perspective, facial biometrics may also aid commercial or political marketing (Davenport et al, 2020). Additionally, governments hope to use the predictive power of AI to identify terrorists or other lawbreakers, using technology to inform policy decisions (Ng, 2017).
Whilst these all seem like promising steps, there are some vital considerations. For example, Apple shares the face mapping data collected on iPhones with third-party app developers, infringing privacy concerns (Statt, 2017). Similarly, Wang & Kosinski (2017) use publicly available profile pictures on dating websites, without explicit consent. There is also no monitoring of who this data is shared with, which poses the risk of identity theft (Lior, 2018).
Wang and Kosinski (2018) claim their research is beneficial to the LGBTQ+ population, as it warns them of predictive models and their possible misuse. The Face ++ algorithm was widely used by the authoritarian Chinese government (Simonite, 2019). Beijing reported its use to help elderly individuals who forget their identity. However, it has been leaked in the NYTimes that Beijing also utilises it to keep a database of marginalised groups (Simonite, 2019; Mozur, 2019). The government identifies every captured Uyghur with Face++ and keeps the data of these individuals forever (Mozur, 2019). From the perspective of consent, one’s facial structures can easily be targeted through security cameras, personal possession of technology, and more. Furthermore, since the ML applications can be highly inaccurate, it is strictly morally impermissible to misgender individuals.
Finally, Wang & Kosinski (2017) present a narrow understanding of sexual orientation, as a simple binary between gay and straight. Not only can this lead to inaccurate results, but it also overlooks centuries of debates regarding the anthropology, sociology, and politics of sexuality. For example, anthropologists have long studied the changes in sexuality throughout an individual’s life course, revealing its fluidity and ability to change (Sandelson, 2018). The authors’ presentation of sexual orientation as a fixed fact points to a lack of engagement with the theory and research topic (Sandelson, 2018).
Discussion
AI can automate pretty much anything in today’s world. Facial recognition software is becoming a key component of our everyday lives. However, this study reveals the core dangers that may arise, namely privacy rights and abusive application for the wrong purposes. It also highlights how intertwined data and politics are. Sexual orientation, alongside other personal traits such as gender or race, are highly political and sociological issues. This study and the emerging debates on algorithms reveal our own biases and social prejudices.
To tackle the pressing concerns that are emerging with the growth of AI, it is crucial for practitioners of different disciplines to work collaboratively. As Lior (2018, 1) puts it, “technology is supposed to enhance our quality of life and, in an ideal world, promote equality.” It is up to us to decide whether this path is worth taking, or whether certain aspects of our lives are worth leaving technologically neutral.
Abdullah, M. F. A., Sayeed, M. S., Sonai Muthu, K., Bashier, H. K., Azman, A., & Ibrahim, S. Z. (2014). Face recognition with Symmetric Local Graph Structure (SLGS). Expert Systems with Applications, 41(14), 6131–6137. https://doi.org/10.1016/j.eswa.2014.04.006
|
Acquisti, A., Brandimarte, L., & Loewenstein, G. (2015). Privacy and human behavior in the age of information. Science, 347(6221), 509–514. https://doi.org/10.1126/science.aaa1465
|
Bjork-James, C. (2017). Bad science journalism: Gay facial recognition. [online] Carwil without Borders.
|
Bollinger, A. (2017). HRC and GLAAD release a silly statement about the ‘gay face’ study. LGBTQ Nation. Retrieved 26 May 2022, from https://www.lgbtqnation.com/2017/09/hrc-glaad-release-silly-statement-gay-face-study/
|
Brown, E., & Perrett, D. (1993). What Gives a Face its Gender? Perception. https://doi.org/10.1068/p220829
|
Davenport, T., Guha, A., Grewal, D. and Bressgott, T. (2019). How Artificial Intelligence Will Change the Future of Marketing. Journal of the Academy of Marketing Science, 48(1), pp.24–42.
|
Deep Neural Networks Are More Accurate Than Humans at Detecting Sexual Orientation From Facial Images | Semantic Scholar. (n.d.). Retrieved 24 May 2022, from https://www.semanticscholar.org/paper/Deep-Neural-Networks-Are-More-Accurate-Than-Humans-Wang-Kosinski/bce12728b74fab15fc154283537a8948c657029a
|
Esteva, A., Kuprel, B., Novoa, R.A., Ko, J., Swetter, S.M., Blau, H.M. and Thrun, S. (2017). Dermatologist-level classification of skin cancer with deep neural networks. Nature, 542(7639), pp.115–118.
|
Jenkinson, J. (1997). Face facts: a history of physiognomy from ancient Mesopotamia to the end of the 19th century. The Journal of Biocommunication, 24(3), pp.2–7.
|
Kavlakoglu, E. (2020). AI vs. Machine Learning vs. Deep Learning vs. Neural Networks: What’s the Difference? [online] IBM.
|
Knight, W. (2017). Biased Algorithms Are Everywhere, and No One Seems to Care. [online] MIT Technology Review.
|
LeCun, Y., Bengio, Y. and Hinton, G. (2015). Deep Learning. Nature, 521(7553), pp.436–444.
|
Levin, S. (2017). New AI can work out whether you’re gay or straight from a photograph. [online] the Guardian.
|
Lior, A. (2018). The Technological ‘Gaydar’ – The Problems with Facial Recognition AI | Yale Journal of Law & Technology. [online] yjolt.org.
|
Marcelino, P. (2018). Transfer learning from pre-trained models. [online] Medium.
|
March 19th, 2018|Featured, research, G., Politics, Science and Comments, T. (2018). The politics of AI and scientific research on sexuality. [online] Engenderings.
|
Martin, D., Carpendale, S., Gupta, N., Hoßfeld, T., Naderi, B., Redi, J., Siahaan, E., & Wechsung, I. (2017). Understanding the Crowd: Ethical and Practical Matters in the Academic Use of Crowdsourcing. In D. Archambault, H. Purchase, & T. Hoßfeld (Eds.), Evaluation in the Crowd. Crowdsourcing and Human-Centered Experiments (Vol. 10264, pp. 27–69). Springer International Publishing. https://doi.org/10.1007/978-3-319-66435-4_3
|
Mattu, J. A., Jeff Larson,Lauren Kirchner,Surya. (n.d.). Machine Bias. ProPublica. Retrieved 7 June 2022, from https://www.propublica.org/article/machine-bias-risk-assessments-in-criminal-sentencing?token=TiqCeZIj4uLbXl91e3wM2PnmnWbCVOvS
|
Miller, A. E. (2018). Searching for gaydar: Blind spots in the study of sexual orientation perception. https://doi.org/10.1080/19419899.2018.1468353
|
Mozur, P. (2019, April 14). One Month, 500,000 Face Scans: How China Is Using A.I. to Profile a Minority. The New York Times. https://www.nytimes.com/2019/04/14/technology/china-surveillance-artificial-intelligence-racial-profiling.html
|
Murphy, H. (2017). Why Stanford Researchers Tried to Create a ‘Gaydar’ Machine (Published 2017). The New York Times. [online] 9 Oct
|
Nahar, K. (2021). Twins and Similar Faces Recognition Using Geometric and Photometric Features with Transfer Learning. IJCDS Journal.
|
Ng, Y.S. (2017). China is using AI to predict who will commit crime next. [online] Mashable.
|
Nissim, K., & Wood, A. (2018). Is privacy privacy? Philosophical Transactions of the Royal Society A: Mathematical, Physical and Engineering Sciences, 376(2128), 20170358. https://doi.org/10.1098/rsta.2017.0358
|
Paul, S., & Acharya, S. K. (2020). A Comparative Study on Facial Recognition Algorithms (SSRN Scholarly Paper No. 3753064). Social Science Research Network. https://doi.org/10.2139/ssrn.3753064
|
Resnick, B. (2018). This psychologist’s ‘gaydar’ research makes us uncomfortable. That’s the point. [online] Vox.
|
Row over AI that ‘identifies gay faces’. (2017, September 11). BBC News. https://www.bbc.com/news/technology-41188560
|
Scherfner, E., Manipula, M., Nisay, A. A., Svitlana, S., Faraguna, C., Grabovskii, P., Sewell, D., & Christiansen, J. (n.d.). Face++—Wiki. Golden. Retrieved 28 May 2022, from https://golden.com/wiki/Face%2B%2B-4N93NWE
|
Scheuerman, M., Wade, K., Lustig, C., & Brubaker, J. (2020). How We’ve Taught Algorithms to See Identity: Constructing Race and Gender in Image Databases for Facial Analysis. Proc. ACM Hum. Comput. Interact. https://doi.org/10.1145/3392866
|
Schutt, R. and O’Neil, C. (2013). Doing Data Science.
|
Simonite, T. (2019). Behind the Rise of China’s Facial-Recognition Giants. Wired. Retrieved 30 May 2022, from https://www.wired.com/story/behind-rise-chinas-facial-recognition-giants/
|
Statt, N. (2017). Apple will share face mapping data from the iPhone X with third-party app developers. [online] The Verge.
|
Strümke, I., & Slavkovik, M. (2022). Explainability for identification of vulnerable groups in machine learning models (arXiv:2203.00317). arXiv. http://arxiv.org/abs/2203.00317
|
The Study of the Negro Problems. (2020). 24.
|
Vasilovsky, A. T. (2018). Aesthetic as genetic: The epistemological violence of gaydar research. https://doi.org/10.1177/0959354318764826
|
Very Engineering Team. (2018). Machine Learning vs Neural Networks: Why It’s Not One or the Other. https://www.verypossible.com/insights/machine-learning-vs.-neural-networks
|
Wang, Y. and Kosinski, M. (2018). Deep Neural Networks Are More Accurate Than Humans at Detecting Sexual Orientation From Facial Images. Stanford Graduate School of Business.
|
Wolmarans, L., & Voorhoeve, A. (2022). What Makes Personal Data Processing by Social Networking Services Permissible? Canadian Journal of Philosophy, 1–16. https://doi.org/10.1017/can.2022.4
|
Wu, W., Protopapas, P., Yang, Z., & Michalatos, P. (2020, July 6). Gender Classification and Bias Mitigation in Facial Images. 12th ACM Conference on Web Science. https://doi.org/10.1145/3394231
|
The LSE Data Science Institute (DSI) acts as the School's nexus for data science, connecting activity across the academic departments to foster research with a focus on the social, economic, and political aspects.
Share your research with the DSI. LSE students can get in touch here.
Note: This article gives the views of the authors, and not the position of the DSI, nor the London School of Economics.