Course details
- DepartmentData Science Institute
- Application codeSS-ME204
Apply
Applications are open
We are accepting applications. Apply early to avoid disappointment.
Overview
Data science has unlocked exciting possibilities for social scientists through its diverse toolkit, including big data analysis, visualisation, and machine learning models, enabling them to extract valuable insights from their data.
Yet, the success of a data-driven project hinges on data quality. This is where data engineering plays a pivotal role. Professionals must ensure that their acquired data is sufficient and accurate and must be adaptable to handle 'messy data' effectively.
A substantial portion of time in data-driven projects (anecdotally 80%) is dedicated to cleaning and pre-processing data, with only 20% said to be devoted to building, evaluating, and deploying machine learning models. Despite the emergence of new AI technologies, which promise to automate many coding tasks, data manipulation is likely to remain an indispensable skill due to the inherent messiness of real-world data.
By the end of this course, you will be proficient in producing a website to communicate your collected data and showcase your newly acquired data-wrangling abilities.
Key information
Prerequisites: Students should already be familiar with computer programming at an introductory level (variables, if-else, loops, functions). We have welcomed complete beginners to this course in the past, and many have done well, but it can be a tough learning curve! We recommend focusing on Python basics if you’d like to prepare in advance. Chapters 1-5 of Automate the Boring Stuff with Python by Al Sweigart is a great starting resource, freely available online.
Level: 200 level. Read more information on levels in our FAQs
Fees: Please see Fees and payments
Lectures: 36 hours
Classes: 18 hours
Assessment: A mid-term problem set (25%) and a final project (75%).
Typical credit: 3-4 credits (US) 7.5 ECTS points (EU)
Please note: Assessment is optional but may be required for credit by your home institution. Your home institution will be able to advise how you can meet their credit requirements. For more information on exams and credit, read Teaching and assessment
Is this course right for you?
This course is ideal for those seeking a hands-on experience with a data science project, whether you want to pursue a career in data science or to experience the data science way of doing things. It is also recommended if you want to strengthen your programming skills. This course will also be relevant if you are starting an MSc or MBA programme of study and wish to learn introductory concepts in the area.
Outcomes
Aims of this course:
Develop the skills to collect public data from the Internet APIs, connect multiple data sources and build websites to report and communicate insights obtained from data.
Learning Objectives:
In this course, you will learn the fundamentals of data engineering, including:
- Understand data structures and formats
- Collect data from websites and APIs
- Apply best practices for efficient data storage
- Create basic SQL queries for data manipulation
- Use Python tools for data preprocessing and reshaping
- Employ AI tools like ChatGPT and GitHub Copilot for coding and debugging
- Organize data into a "tidy" format suitable for analysis
- Conduct exploratory data analysis with static and dynamic visualisations
- Create simple websites to report findings effectively
Content
Faculty
The design of this course is guided by LSE faculty, as well as industry experts, who will share their experience and in-depth knowledge with you throughout the course.
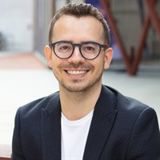
Dr Jonathan Cardoso-Silva
Assistant Professor (Education)
Department
The Data Science Institute (DSI) forms the institutional cornerstone of data science activity at the London School of Economics and Political Science. Working alongside the academic departments across the School, the DSI's mission is to foster the study of data science and new forms of data with a focus on their social, economic, and political aspects.
The DSI aims to host, facilitate and promote research in social and economic data science through an annual programme of seminars, workshops and research projects delivered by a range of academic experts and research students.
Join our mailing list
Sign up to get more information
Apply
Applications are open
We are accepting applications. Apply early to avoid disappointment.