Course details
- DepartmentDepartment of Methodology
- Application codeSS-ME202
Apply
Applications are open
We are accepting applications. Apply early to avoid disappointment.
Overview
This course focuses on data about connections, forming structures known as networks. Networks and network data describe an increasingly vast part of the modern world, through connections on social media, communications, financial transactions, and other ties.
This course covers the fundamentals of network structures, network data structures, and the analysis and presentation of network data. Students will work directly with network data, and structure and analyse these data using R.
Social networks have always been at the centre of human interaction, but especially with the explosive growth of the internet, network analysis has become increasingly central to all branches of the social sciences. How do people influence each other, bargain with each other, exchange information (or germs), or interact online? A diverse array of deep questions about human behaviour can only be answered by examining the social networks encompassing and shifting around us. Network analysis has emerged as a cross-disciplinary science in its own right, and has in fact proven to be of even greater generality and broader applicability than just the social, extending to ecology, physics, genetics, computer science, and other domains.
This course will examine the key papers in the development of social network analysis, and will develop the theory and methodological tools needed to model and predict social networks and use them in social sciences as diverse as sociology, political science, economics, health, psychology, history, or business. The core of the course will comprise the essential tools of network analysis, from centrality, homophily, and community detection, to random graphs, network formation, and information flow. Alongside this we will read a series of substantive and seminal papers, shaped in part by the interests of the students and their various backgrounds, with a particular focus on the difficult task of causal inference in social networks. The course will also provide an introduction to network modelling, analysis and visualisation using R.
Key information
Prerequisites: An introductory course in probability or statistics is a requirement for the course. Prior experience with R is helpful, but is not a requirement (we will cover the basics of programming in R as part of the course).
Level: 200 level. Read more information on levels in our FAQs
Fees: Please see Fees and payments
Lectures: 36 hours
Classes: 18 hours
Assessment: Two examinations - one mid-session examination (30%) and a final examination (70%), both examinations will be take-home and open book.
Typical credit: 3-4 credits (US) 7.5 ECTS points (EU)
Please note: Assessment is optional but may be required for credit by your home institution. Your home institution will be able to advise how you can meet their credit requirements. For more information on exams and credit, read Teaching and assessment
Is this course right for you?
This course is ideal if you are wanting to get a hands-on introduction to how we can represent, describe, and model social networks. If you are looking to build your skills in data science, while still having a strong grounding in social theory, you should consider this course.
Given the breadth of applicability of network analysis, this could be of interest if you are looking to pursue further study with an MSc or MBA, or turning to a career in consulting or management.
Outcomes
- Construct and describe empirical network data.
- Identify the main analytical reasons for tailored network analysis tools.
- Recognize, implement, and interpret the main analytical tools for describing and modeling social network data.
- Critically approach novel network datasets and analyses.
Content
Faculty
The design of this course is guided by LSE faculty, as well as industry experts, who will share their experience and in-depth knowledge with you throughout the course.
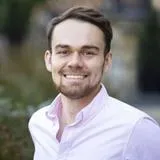
Dr Patrick Gildersleve
Visiting Lecturer
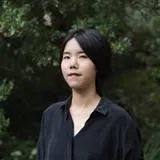
Dr Linda Li
LSE Fellow
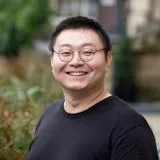
Dr Yuanmo He
LSE Fellow
Department
LSE’s Department of Methodology is an internationally recognised centre of excellence in research and teaching in the area of social science research methodology. The disciplinary backgrounds of the staff include political science, statistics, sociology, social psychology, anthropology and criminology. The Department coordinates and provides a focus for methodological activities at the School, providing methods training to students from across the School.
With the training in the core social scientific tools of analysis and research offered by the Department of Methodology, coupled with its numerous workshops in other transferable skills such as computer programming and the use of methods-related software, the Department of Methodology ensures that the School’s students and staff have the expertise and training available to maintain the School’s excellence in social scientific research. We also work closely with colleagues in the Departments of Statistics and Mathematics to cover advanced topics, including in the interdisciplinary area of social applications of data science.
Join our mailing list
Sign up to get more information
Apply
Applications are open
We are accepting applications. Apply early to avoid disappointment.